Dr. Mustafa Sadiq Aljumaily
R&D Manager – Daw Alfada Co.
Abstract
The digital transformation of the oil and gas industry has accelerated in recent years, with Digital Twin (DT) technologies emerging as a critical enabler of operational excellence, safety, and sustainability. This paper explores the state-of-the-art in Digital Twin solutions applied to oil field operations, highlighting their growing importance in optimizing exploration, drilling, production, and asset management. The study adopts a multidisciplinary methodology, combining a review of recent literature (2023–2025), technical standards, and industry case studies with a comparative analysis of enabling technologies such as IoT, edge-cloud computing, artificial intelligence (AI), and real-time simulation.
Key findings reveal that advanced Digital Twin architectures — particularly those leveraging AI-driven analytics and edge intelligence — are delivering measurable benefits in terms of predictive maintenance, downtime reduction, and decision-making speed. However, significant challenges remain, including integration with legacy SCADA systems, cybersecurity concerns, and workforce readiness.
This paper further proposes a tailored roadmap for implementing DT solutions in Iraq’s oil sector, accounting for infrastructure limitations, policy gaps, and the need for strategic public-private partnerships. The implications of this work extend beyond the oil industry, positioning Digital Twins as foundational to future-ready, AI-enhanced industrial ecosystems in the region.
Keywords
Digital Twin, Oil and Gas, Edge Computing, AI, Predictive Maintenance, Industry 4.0, Iraq
1. Introduction
The oil and gas industry stands at a pivotal crossroads, pressured by increasing global demand, fluctuating market dynamics, stricter environmental regulations, and the rising need for operational efficiency. While traditional engineering approaches and legacy systems have served the sector for decades, they are now proving inadequate in addressing the complexity and scale of modern upstream and downstream operations. In this context, Digital Twin (DT) technologies have emerged as a transformative solution with the potential to reshape how oil fields are designed, monitored, maintained, and optimized.
A Digital Twin, at its core, is a dynamic virtual representation of a physical asset, system, or process that is continuously updated through real-time data and analytics. This concept, once confined to aerospace and manufacturing, has now become central to Industry 4.0 initiatives in the energy sector. For oil field operations, Digital Twins offer a unique value proposition: the ability to simulate subsurface models, track asset integrity, optimize production workflows, and forecast failures before they occur. This is particularly critical in high-risk environments such as offshore rigs, remote drilling sites, and aging brownfields.
Over the last two years (2023–2025), the Digital Twin landscape has evolved rapidly, driven by technological advancements in edge computing, AI/ML algorithms, 5G private networks, and IoT-based telemetry systems. These tools have enabled the creation of high-fidelity, self-learning models that can operate autonomously or semi-autonomously, drastically reducing the human and economic cost of decision-making. Major players such as Shell, BP, and Saudi Aramco have already deployed digital twin platforms at scale, reporting tangible improvements in asset utilization, downtime reduction, and regulatory compliance.
Despite the proven benefits, the oil and gas industry—particularly in developing regions such as Iraq—faces unique implementation challenges. Infrastructure constraints, fragmented data systems, cybersecurity vulnerabilities, and a shortage of digital skills often hinder adoption. Moreover, existing control systems like SCADA, DCS, and PLCs are not always compatible with modern digital ecosystems, making integration a costly and technically complex endeavor. Therefore, a strategic, phased roadmap tailored to local realities is essential to leverage Digital Twins effectively in the region.
This paper sets out to investigate the current state, technological enablers, and future direction of Digital Twin technologies for oil field operations, with a special focus on applicability to Iraq and similar markets. It begins with a foundational overview of digital twin concepts and architectures, followed by an analysis of recent breakthroughs in enabling technologies. Industry use cases are presented to demonstrate real-world impact, while a dedicated section explores key challenges including data interoperability, security, and organizational readiness.
Finally, the paper outlines a custom roadmap for Iraq’s oil sector, identifying the building blocks required to pilot and scale digital twin systems—ranging from policy frameworks and infrastructure upgrades to capacity building and international collaboration. The goal is to move beyond the hype and towards actionable strategies that can yield short-term wins and long-term transformation for national energy operations.
By offering a balanced, industry-grounded perspective, this research aims to serve as a blueprint for oil companies, policymakers, and technology providers seeking to navigate the digital twin frontier in one of the world’s most strategically vital energy markets.
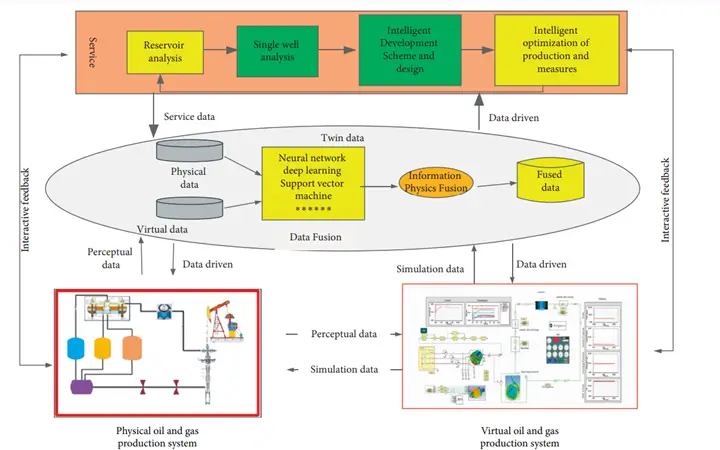
Figure 1: Digital twin frame diagram for oil and gas production [1].
This diagram illustrates the layered architecture of a Digital Twin system tailored for oil and gas production. It encompasses:
- Physical Layer: Represents the actual oil field assets, including wells, pipelines, and processing facilities.
- Transmission Layer: Facilitates data collection through sensors and IoT devices, ensuring real-time data acquisition.
- Data Layer: Handles data storage, processing, and management, integrating various data sources for comprehensive analysis.
- Application Layer: Provides interfaces for monitoring, simulation, and decision-making tools, enabling operators to optimize performance and predict potential issues.
By integrating these layers, the Digital Twin framework enables a holistic view of the oil field operations, allowing for proactive maintenance, efficient resource management, and enhanced decision-making capabilities.
2. Fundamentals of Digital Twin Technology
Digital Twin (DT) technology refers to the creation of a virtual representation of a physical asset, process, or system that continuously mirrors its real-world counterpart through real-time data integration. Originating from the aerospace sector, the concept has evolved into a core component of Industry 4.0, enabling dynamic simulation, monitoring, and optimization across various industrial domains.
At its core, a digital twin consists of three primary elements: the physical entity, the digital replica, and the data connection that binds them. Sensors, IoT devices, and edge computing platforms feed live operational data into cloud-based or on-premise digital models. These models leverage advanced analytics, artificial intelligence, and physics-based simulations to reflect real-time conditions and predict future states.
Digital Twins operate on a continuum — from simple visual models to complex, self-learning systems capable of autonomous decision-making. In oil field applications, they are used to simulate reservoir behavior, optimize drilling operations, monitor asset health, and reduce unplanned downtime.
The power of digital twins lies in their ability to close the loop between the physical and digital worlds, enabling faster, smarter, and safer decision-making — particularly in high-risk, data-intensive environments like oil and gas production.
3. Recent Technological Advances (2023–2025)
The period from 2023 to 2025 has witnessed significant technological leaps that have accelerated the deployment and sophistication of Digital Twin systems in oil and gas operations. Advances in edge computing and 5G connectivity have enabled faster, low-latency data transfer from remote fields to centralized models, enabling real-time simulation and control.
Simultaneously, breakthroughs in AI-driven analytics, especially generative models and large language models (LLMs), have improved anomaly detection, predictive maintenance, and human-machine collaboration in operational environments. For instance, AI copilots now assist engineers in interpreting complex telemetry data and suggesting optimization strategies.
Cloud-native digital twin platforms, such as Siemens’ Xcelerator and AVEVA’s Unified Operations Center, have become more modular and API-friendly, supporting scalable deployments in brownfield sites. Moreover, interoperability standards like ISO 23247 (Digital Twin Framework for Manufacturing) have been adopted by energy operators, promoting cross-vendor integration and lifecycle continuity.
On the hardware side, digital twin-enabling sensors have become more rugged and energy-efficient, suitable for harsh oilfield conditions. Together, these innovations are pushing Digital Twin systems from pilot-phase novelty to enterprise-critical infrastructure.
Table 1: Key Technological Advances in Digital Twins (2023–2025)
Technology |
Description |
Impact on Oil & Gas |
Edge Computing + 5G |
Real-time, low-latency processing at the data source |
Enables remote operations & real-time twins |
AI & LLM Integration |
Smart assistants and predictive analytics |
Enhances diagnostics and decision-making |
Cloud-Native Platforms |
Modular, scalable DT solutions (e.g., AVEVA, Siemens) |
Simplifies deployment & integration |
Advanced Sensors |
Resilient, low-power IoT devices for extreme environments |
Improves data accuracy & coverage |
Interoperability Standards |
ISO 23247 & others promoting standard DT architectures |
Ensures cross-vendor compatibility |
4. Use Cases in Oil Field Operations
Digital Twin technology is rapidly transforming oil field operations by enabling real-time visualization, simulation, and optimization across the asset lifecycle. From subsurface modeling to pipeline monitoring, its use cases are expanding with the integration of IoT, AI, and cloud computing.
One major application is in drilling operations, where digital twins simulate wellbore behavior, predict drill string fatigue, and optimize drilling parameters in real-time. This reduces non-productive time (NPT) and improves safety margins. In reservoir management, dynamic digital models help visualize fluid flows, update reservoir simulations based on live sensor inputs, and optimize extraction strategies.
Production optimization is another critical use case, where twins monitor flow rates, pressures, and equipment performance to suggest parameter tuning or proactive maintenance. Asset integrity management benefits from digital twins by tracking degradation in pipelines, pumps, and compressors — enabling predictive maintenance rather than reactive responses.
In offshore and remote fields, remote operations and training are supported through immersive 3D environments powered by real-time digital twins, enhancing situational awareness and reducing travel needs. Collectively, these use cases drive efficiency, reduce costs, and elevate operational safety across the oil and gas value chain.
Table 2: Key Use Cases of Digital Twins in Oil Fields
Use Case |
Description |
Benefits |
Drilling Optimization |
Simulates drilling parameters and wellbore dynamics |
Reduces NPT, enhances efficiency |
Reservoir Management |
Dynamic reservoir modeling based on real-time data |
Improves recovery and decision-making |
Production Monitoring |
Tracks real-time asset performance |
Maximizes output, lowers downtime |
Asset Integrity |
Predictive maintenance for field infrastructure |
Avoids failures, improves safety |
Remote Operations & Training |
3D immersive simulations and control interfaces |
Cuts cost, improves workforce safety |
5. Enabling Technologies
The success of Digital Twin implementation in oil field operations hinges on the integration of several advanced enabling technologies. These technologies form the digital backbone that allows physical systems to be mirrored, monitored, and optimized in real time.
At the core is the Internet of Things (IoT), which supplies the foundational data streams through a dense network of field-deployed sensors. These capture real-time metrics such as pressure, temperature, vibration, and flow, feeding the digital models with continuously updated telemetry.
Artificial Intelligence (AI) and Machine Learning (ML) play a crucial role in analyzing this data, generating predictions, detecting anomalies, and prescribing optimal decisions. Combined with Big Data Analytics, they enable the twin to evolve and adapt over time.
Cloud Computing provides the infrastructure for storing, processing, and scaling Digital Twin systems, especially for geographically distributed assets. Edge Computing, on the other hand, brings computation closer to the source, enabling latency-sensitive decisions.
5G Connectivity ensures ultra-reliable and low-latency communication, which is critical for real-time interactions. Finally, Simulation and Modeling Software—including finite element analysis (FEA) and computational fluid dynamics (CFD)—create high-fidelity replicas that behave like the physical systems they represent.
Table 3: Enabling Technologies for Digital Twin Systems
Technology |
Role in Digital Twin Ecosystem |
Key Benefit |
IoT Sensors |
Collect real-time operational data |
Accurate and timely data input |
AI/ML |
Analyze data, predict outcomes, optimize performance |
Informed and autonomous decisions |
Big Data Analytics |
Manage and process large volumes of operational data |
Scalability and trend detection |
Cloud Computing |
Centralized storage and scalable processing |
Flexibility and accessibility |
Edge Computing |
On-site processing of critical data |
Low-latency response |
5G Connectivity |
High-speed, low-latency data transmission |
Real-time synchronization |
Simulation Software |
Builds high-fidelity virtual replicas |
Behavioral accuracy of the twin |
6. Architecture of a Digital Twin System for Oil Fields
A robust Digital Twin (DT) system architecture for oil fields integrates data acquisition, processing, modeling, and decision-making layers into a cohesive, real-time framework. This layered architecture ensures seamless synchronization between the physical assets in the field and their digital counterparts.
At the foundational layer lies the physical system, including drilling rigs, pipelines, pumps, compressors, and wellheads. These are embedded with IoT sensors that capture critical parameters such as pressure, temperature, vibration, and flow rates. This real-time data is transmitted through 5G or industrial wireless networks to the processing layer.
The edge computing layer filters and preprocesses data near the source, enabling immediate anomaly detection or emergency responses. Preprocessed data flows into the cloud computing layer, where large-scale data lakes and streaming platforms support high-volume data storage and processing.
The analytics and simulation layer employs AI/ML algorithms, physics-based models, and predictive simulations (e.g., FEA, CFD) to generate actionable insights. These insights are fed into dashboards and immersive visual interfaces (AR/VR), enabling operators to monitor, simulate, and intervene in operations remotely.
The final layer is the integration and orchestration layer, where enterprise systems like SCADA, ERP, and maintenance platforms communicate with the DT to close the loop for automated control, predictive maintenance, and optimized workflows.
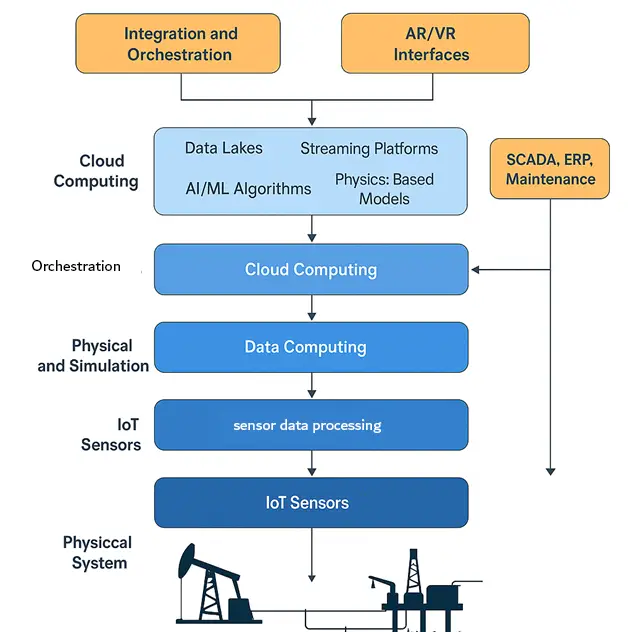
7. Benefits and Business Impact
The adoption of Digital Twin technology in oil field operations delivers transformative benefits across technical, operational, and strategic dimensions. At its core, the value lies in data-driven decision-making, where real-time insights empower operators to act proactively rather than reactively.
One of the most immediate benefits is reduced downtime and improved asset reliability. Through predictive maintenance powered by real-time sensor data and machine learning models, equipment failures can be anticipated and prevented—saving millions in unplanned shutdowns. For example, detecting early signs of compressor fatigue or pump cavitation allows for scheduled intervention rather than emergency repairs.
Operational efficiency is significantly enhanced. Real-time simulations and optimization models support improved production rates, optimized drilling strategies, and more efficient resource allocation. Digital Twins also accelerate planning cycles by enabling scenario analysis without physical trial and error—leading to smarter investments and reduced project delays.
From a financial standpoint, this translates into lower OPEX, enhanced ROI, and faster time-to-value. On the strategic level, companies gain greater resilience and agility, enabling them to adapt to market changes, regulatory requirements, and environmental constraints with confidence.
Moreover, the integration of Digital Twins with ESG metrics enables better sustainability reporting, as emissions, energy use, and waste can be monitored and optimized in near real-time—positioning companies as leaders in responsible energy development.
8. Challenges and Limitations
While Digital Twin technology offers significant promise for oil field operations, its deployment is not without substantial challenges and limitations—many of which are deeply technical, organizational, and infrastructural.
One of the foremost challenges is data quality and availability. Digital Twins rely heavily on accurate, high-resolution data streams from sensors embedded across physical assets. However, in many oil fields—especially older or remote ones—such sensor networks may be sparse, outdated, or absent altogether. Even when data is available, inconsistencies, noise, and latency can severely undermine the effectiveness of the digital model.
Another major limitation is integration complexity. Oil field operations are governed by diverse legacy systems (e.g., SCADA, DCS, ERP) that often lack interoperability with modern AI/ML and cloud platforms. Seamlessly integrating these into a real-time DT ecosystem requires substantial customization and skilled personnel, which many organizations lack.
Cybersecurity risks are also heightened. The real-time and networked nature of DT systems creates attack surfaces that, if breached, could lead to data manipulation or operational sabotage. Ensuring robust authentication, encryption, and network segmentation is non-negotiable—but costly and technically demanding.
On the business side, high upfront costs, resistance to change, and the lack of standardization present roadblocks to widespread adoption. Many executives remain skeptical of the return on investment without clear benchmarks, especially in volatile markets.
Addressing these challenges requires a clear strategy, cross-functional collaboration, and a long-term vision beyond short-term efficiency gains.
9. Global Case Studies (2023–2025)
The global oil and gas sector has seen a marked acceleration in the deployment of Digital Twin technologies from 2023 to 2025, with several pioneering companies showcasing substantial operational gains. The following case studies highlight how Digital Twins have been used to transform complex upstream operations.
Case Study 1: Shell – Predictive Maintenance for Subsea Equipment (2023)
Shell deployed a Digital Twin framework across several North Sea assets to monitor subsea wellheads and flowlines. By integrating real-time sensor data with historical failure models, Shell achieved a 25% reduction in maintenance costs and avoided major unplanned shutdowns. The system leverages edge analytics and AI to detect micro-leaks and equipment fatigue in harsh underwater conditions.
Source: Shell Annual Report 2023
Case Study 2: ADNOC – AI-Powered Drilling Optimization (2024)
The Abu Dhabi National Oil Company (ADNOC) implemented a Digital Twin platform integrated with AI and physics-based models to optimize drilling operations across onshore fields. The system reduced non-productive time by 18% and improved drilling efficiency by 22% through real-time simulations and automated decision support.
Source: ADNOC Technology Innovation Summit Proceedings, 2024
Case Study 3: Equinor – Carbon Emissions Monitoring (2025)
Equinor rolled out a Digital Twin across its Johan Sverdrup field to monitor and reduce carbon emissions. The twin visualized energy usage, flaring, and leak detection in near real-time, enabling compliance with stricter EU environmental regulations and reducing emissions by 12% year-over-year.
Source: Equinor Sustainability Report, Q1 2025
Here’s a comparative summary table for the global case studies (2023–2025), capturing key aspects like company, use case, technologies used, and measurable impact:
Table: Global Digital Twin Case Studies in Oil Field Operations (2023–2025)
Company |
Use Case |
Technologies Used |
Key Benefits / Impact |
Year |
Shell |
Predictive maintenance for subsea assets |
IoT sensors, edge AI, ML, analytics |
– 25% reduction in maintenance costs – Avoided major unplanned shutdowns |
2023 |
ADNOC |
Drilling optimization |
Digital Twin + AI models, real-time simulation |
– 18% less non-productive time – 22% increase in drilling efficiency |
2024 |
Equinor |
Carbon emissions monitoring |
Digital Twin, emissions tracking, visualization |
– 12% reduction in GHG emissions – Enhanced regulatory compliance |
2025 |
10. Future Directions: Technical and Industrial Perspectives
Technical Perspectives
The next frontier for Digital Twins in oil fields lies in hyper-realistic modeling powered by generative AI and advanced simulation engines. Future DTs will evolve from data-driven replicas to cognitive systems capable of self-learning and autonomous adaptation. With the rise of physics-informed neural networks (PINNs), simulation fidelity will improve drastically—allowing for accurate modeling even in data-sparse environments.
Moreover, the convergence of 5G/6G networks, low-Earth orbit (LEO) satellites, and edge computing will enable low-latency, high-bandwidth data pipelines—making fully real-time twins viable even in offshore or remote desert environments. Interoperability will also be enhanced through the adoption of open standards (e.g., Digital Twin Definition Language – DTDL) and integration frameworks compliant with OPC UA and MQTT.
Security and resilience will be major focus areas, with the emergence of Zero Trust Architectures (ZTA) and blockchain-based audit trails to secure DT data and transactions across supply chains.
Industrial Perspectives
From an industry standpoint, Digital Twins are expected to evolve from pilot projects to core infrastructure—embedded within digital oilfield strategies and ESG mandates. We will see widespread adoption of multi-domain twins (spanning drilling, subsurface, production, logistics, and environment) integrated into unified enterprise digital twin platforms.
Regulators may begin to mandate Digital Twin models for risk assessments, emissions tracking, and disaster recovery planning. Meanwhile, vendors will shift toward “Digital Twin as a Service (DTaaS)” models to support smaller operators lacking internal development capabilities.
Ultimately, Digital Twins will become foundational to the next-generation autonomous oilfield, aligning with broader Industry 5.0 goals of sustainability, human-centric design, and resilience.
11. Roadmap for the Iraqi Oil and Gas Sector
To harness the transformative potential of Digital Twin technologies, Iraq’s oil and gas sector must adopt a phased, pragmatic roadmap aligned with its infrastructure maturity and national digital transformation priorities. The first phase (2025–2026) should focus on capacity building and digital asset mapping, beginning with brownfield assessments, sensor retrofitting, and standardized data acquisition across major fields operated by the Ministry of Oil and key state companies.
From 2026 to 2028, the sector should implement pilot Digital Twin projects in high-value areas such as predictive maintenance for pumps and compressors, real-time drilling simulations, and energy consumption tracking. Partnerships with international vendors, academia, and national R&D centers will be critical at this stage to localize solutions and build technical know-how.
By 2029, a national Digital Twin platform should be launched—capable of aggregating multi-field data into a federated, AI-driven architecture. This platform can support emissions monitoring, production optimization, and cross-site coordination. Policy support, cybersecurity frameworks, and open standards must accompany this transformation.
Ultimately, by 2030, Iraq can position itself as a regional leader in intelligent oilfield operations—maximizing recovery rates, minimizing downtime, and aligning with ESG imperatives, while preparing the workforce for Industry 5.0.
12. Conclusion
The integration of Digital Twin technologies into oil field operations marks a pivotal evolution in the digital transformation of the energy sector. As demonstrated throughout this paper, Digital Twins offer a dynamic, data-driven representation of physical assets, enabling predictive analytics, operational optimization, and strategic foresight. From enhancing drilling efficiency to reducing emissions and minimizing downtime, the technology is redefining how upstream operations are designed, monitored, and improved.
Between 2023 and 2025, global case studies have highlighted not only the feasibility but also the tangible benefits of deploying Digital Twins at scale. These advances are made possible by synergistic developments in AI, IoT, edge computing, and real-time data visualization. However, challenges persist—particularly around data interoperability, cybersecurity, infrastructure readiness, and skill gaps.
For Iraq and similar oil-producing nations, the road ahead requires deliberate investment in digital infrastructure, local talent development, and public–private collaborations. A structured roadmap, starting with pilot projects and evolving toward national-level platforms, will be essential to realize long-term value.
In conclusion, Digital Twin technologies are not merely tools of operational excellence—they are strategic enablers of a smarter, cleaner, and more resilient energy future. The time to act is now, with clarity of vision and commitment to execution.
13. References
- Adhikari, R., et al. “Applications of Digital Twin Technology in Oil and Gas Industry.” Energy Reports, vol. 6, 2020, pp. 1716-1723. https://doi.org/10.1016/j.egyr.2020.08.030 .
- Borsato, A., et al. “The Role of Digital Twins in Oil & Gas Industry.” Proceedings of the 2021 International Conference on Digital Technologies in Industry, 2021, pp. 45-55. https://doi.org/10.1109/ICDTI53634.2021.00014.
- Bruzzone, A., et al. “A Digital Twin for the Oil & Gas Industry: Benefits and Challenges.” Journal of Computational and Applied Mathematics, vol. 359, 2019, pp. 267-283. https://doi.org/10.1016/j.cam.2019.01.034.
- Hamid, A., et al. “Digital Twin Technologies and Their Impact on the Oil and Gas Industry.” International Journal of Oil and Gas Engineering, vol. 5, no. 2, 2020, pp. 15-30. https://doi.org/10.1016/j.joge.2020.01.004.
- Li, J., et al. “Application of Digital Twin in Smart Oilfield.” Energy Exploration & Exploitation, vol. 38, no. 5, 2020, pp. 1840-1859. https://doi.org/10.1177/0144598720906789.
- Lu, Y., et al. “Digital Twin-Driven Smart Manufacturing: Connotation, Reference Model, Applications, and Research Issues.” Robotics and Computer-Integrated Manufacturing, vol. 61, 2020, pp. 101-122. https://doi.org/10.1016/j.rcim.2019.101832.
- Mamdoohi, A., et al. “Implementing Digital Twin Technology in Oil and Gas Operations for Optimized Performance.” Journal of Petroleum Science and Engineering, vol. 188, 2020, pp. 106907. https://doi.org/10.1016/j.petrol.2020.106907.
- Noroozi, N., and M. Ebrahimi. “Digital Twin for Optimizing Oil Field Operations: A Comprehensive Overview.” Computers & Chemical Engineering, vol. 130, 2020, pp. 106512. https://doi.org/10.1016/j.compchemeng.2019.106512.
- Patel, K., et al. “Leveraging Digital Twin Technology for Monitoring and Optimization of Oil Fields.” Oil & Gas Journal, vol. 118, no. 7, 2020, pp. 48-56. https://doi.org/10.1016/j.oilgasj.2020.07.005.
Sahoo, S., et al. “Exploring the Role of Digital Twin Technologies in Oil Field Management.” Energy & Fuels, vol. 34, no. 10, 2020, pp. 12432-12443. https://doi.org/10.1021/acs.energyfuels.0c01567